Data analytics is the process of transforming, cleansing, and modeling data.
This fast-growing industry continues to innovate and evolve, and you might find yourself coming across terms that seem unfamiliar when describing data analytics.
These data glossary terms are a resource to help guide you through the keywords most commonly used to help transform your vocabulary to be an analytics all-star!
Starting with the four types of analytics and types of data, we will walk through the terms to familiarize you with and evolve your understanding of data.
Refer to this blog frequently as I will be adding to and updating more terms to the glossary.
Sections:
- Introduction
- The Four Types of Analytics
- Types of Data
- Data Governance, Quality, Strategy, Modeling
- Glossary Terms
- Iteration Insights
- Conclusion
Definitions For The Four Types of Analytics
To understand the importance of analytics to your organization, you must first understand the type of analytics themselves.
Analytics are pivotal in decision-making and create valuable insights for organizations. Knowing the types of analytics and their value will help guide you in various aspects of your business and analytics journey.
The four types of analytics are:
Descriptive Analytics, Diagnostic Analytics, Predictive Analytics, and Prescriptive Analytics.
1. Descriptive Analytics
This type of analytics is used when assessing historical data to answer the fundamental question of “What happened?”. Looking at patterns of past events, we can identify specific patterns that are predicted to occur again within the data.
Descriptive analytics is often visualized as Pie charts, bar charts, tables, or line graphs.
2. Diagnostic Analytics
A form of advanced analytics that examines data or content to answer the question, “Why did it happen?”. It is characterized by techniques such as drill-down, data discovery, data mining, and correlations this usually occurs after an organization has achieved descriptive analytics.
3. Predictive Analytics
Another type of advanced analytics that uses data and information to answer the question “What is likely to happen?”.
Predictive analytics is impactful for organizations that require large amounts of high-quality data. This technique involves forecasting, multivariate statistics, pattern matching, predictive modeling, and regression analysis.
4. Prescriptive Analytics
The final level and most advanced level of analytics is prescriptive analytics. This method analyzes analytics to determine “What should be done?”.
These insights rely not only on the quality of your analytics but also the accuracy to ensure that organizations can make well-thought decisions to achieve desired results and to increase revenue.
Types of Data
Big Data
Refers to large amounts of data collected from various platforms in real-time that are analyzed. Big data uses components of AI through machine analyses to understand data and information to be most efficient.
Master Data
Foundational data is stored and centralized across all aspects of the business. Master data is used across multiple systems and processes of your business functions. This is the most common data linked to all other data sets within your organization.
Transactional Data / Event Data
This data type is utilized to record the transactions of the data across various functions. The data is forecasted to predict business events and the business transactions to follow and to record instances of change.
Transactional data is monitored and updated on an ongoing basis. This data can be categorized into product segments, customer segments, and price.
Data Governance, Modeling, Quality, Strategy
Data Governance
A set of practices including both monitoring and planning data to ensure it is useful as a strategic asset. The goal is to ensure confidence in the data for its use.
Dimensional Modelling
The final step of structuring your data involves developing context to the data adding value and insights to the data user. Identifying and categorizing the data will then be presented in the form of tables.
Data Quality
The data is consistent and reliable to ensure accuracy in providing useful insights to the data consumer and is “fit for use.”
Data Strategy
The set of decisions and actions that address how your organization will solve the critical pieces of data that support your business goals.
Some questions to ask when developing your data strategy include:
- Am I spending my investment the right way?
- Is this the proper technology we should be using?
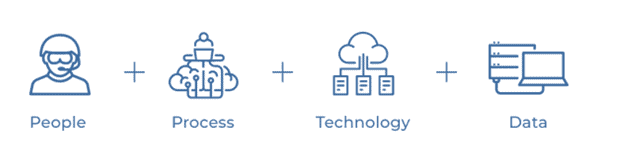
Glossary Terms
Central Version of the Truth
An established understanding of data that is used company-wide. It ensures that sales, marketing, finance, and other departments are using the same data sets, tooling, and definitions to reach various business decisions.
Data Competency
Acquiring and adapting knowledge around data including the proper certifications and qualifications to excel in both knowing and mastering data.
Data Culture
Developing your data to make an impact and evolving it to utilize it to create value. This means data within your organization can be used on a day-to-day basis to improve productivity.
Data-Driven Culture
Companies’ business decisions are based on evidential data to make them more accessible and usable across various business functions.
Data Engineer
Works in the back end with the data through gathering, consolidating, and modeling the data. Data engineers oversee the management, monitoring, security, and privacy of the data so that is prepared for the data user.
Data Foundation
Showcasing the value of your data through adding AI insights to process and model the data. The first step of the data foundation is to gather and have all available data. The data is then strengthened by adding models and reports and developing a strategy to increase its value to the data user.
Data Literacy
Becoming fluent in the data to provide insights and interpret the data. The ability to read, assess and answer questions about the data to develop a full-depth critical-thinking mindset for better decision-making capabilities across the organization.
Data Preparation
Ensuring that data is ready for analysis. This includes removing columns, replacing nulls in cells, updating missing values, and merging tables.
These transformations are necessary to make data ready for reporting.
Data Scientist
Apply advanced analytics to the data using Statistics, AI, machine learning to make it more valuable for decision making and interpretation.
People, Process, Technology, and Data
Dealing with the data user processing the data. Creating the processes within the technology used and creating a balance within all the parts of people, process, technology, and data.
Reporting
Using visuals for analysis to represent insights from the data makes it more comprehensive to the data user. This collection of data makes it more understandable for decision-makers to interpret and define meaning to their organization and data.
Self-Serving Data
Making data easy to utilize by the data user themselves to create and establish their analysis of the data. This includes adapting the ability to modify and adapt decisions based on your reports.
Star Schema Methodology
Creates capabilities for the data used to filter and restructure their transactional database including creating data warehouses, through fact tables to link and find relationships across the data sets.
Storytelling
Communicating data to decision-makers through visualizations to better understand the data to all its users. Storytelling with data makes it more useful and engaging to better understand decision-makers.
Iteration Insights
Iteration Insights is tech-enabled data analytics company based in Calgary, Alberta. Our goal is to empower people with data by enhancing skill sets and experience to understand the processes of data. We ensure our clients get the most usage of their data and insights while offering top-tier knowledge, expertise, and training to our people.
One of our core values is life-long learning. We strive for this by offering a wide range of training, user groups, and thought-driven content to help enhance digital modernization journeys.
Whether you are just starting on your analytics journey or are looking to enhance it, we offer hands-on instructor-led training that can help you on your journey to becoming an analytics superhero!
If you are new to analytics and looking to adapt your knowledge, our Introduction Course is a great way to start learning data analytics and creating dashboards in Power BI!
Those more familiar with the analytics space and are looking to level up on their knowledge of Power Query, Dax, and Data Modeling are strong candidates for our Intermediate Training.
For a full list of events and upcoming training dates, check Iteration Insight’s Events Page.
Conclusion
Data and analytics is a complex and multi-functional topic. As a Marketing Coordinator, I work in the analytics industry, and I am learning every day of the new terms, processes, and functions of data. This blog is a resource to develop an understanding of data analytics and to become more familiar with the industry terms. Refer to this glossary, as I will continue to update with information and terminology!